
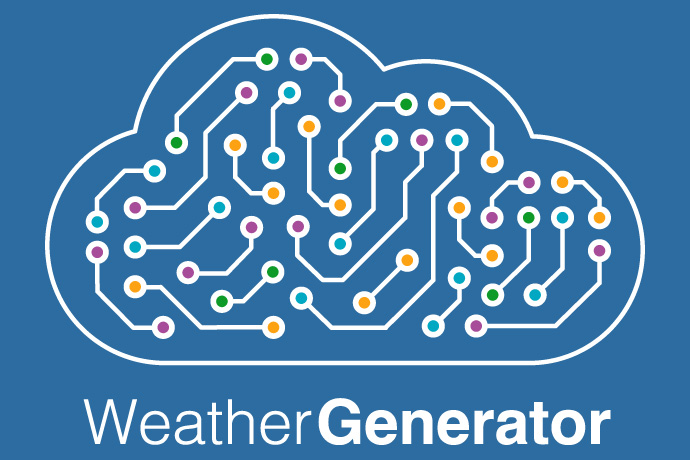
ECMWF is coordinating an EU Horizon project called WeatherGenerator which aims to use machine learning in novel ways for weather forecasting and to model related Earth system processes.
The developments in the WeatherGenerator will feed into the digital twins implemented by ECMWF in the EU’s Destination Earth (DestinE) initiative, in which we are one of three entrusted entities alongside ESA and EUMETSAT. It is anticipated that it can also be used to supplement the Centre’s standard weather forecasts.
The WeatherGenerator is being developed by 16 European organisations, in a four-year EU-funded initiative which is to start in February 2025. Participants include the national meteorological services of several ECMWF Member and Co-operating States, and research centres active in high-performance computing, machine learning, and Earth system modelling. ECMWF is currently looking to fill four positions related to the project.
A broad application
The fundamental idea of the WeatherGenerator is to build one machine learning tool that can be used and adapted for a large number of specific tasks. That is why the WeatherGenerator will be a ‘foundation model’.
In this way, it is different from ECMWF’s current Artificial Intelligence Forecasting System (AIFS), which is designed specifically for weather forecasting.
In addition to a broad range of applications in the field of weather and climate prediction, the WeatherGenerator is also intended to be used for renewable energy and flood prediction and in the areas of food security, health and the biosphere (see the figure).
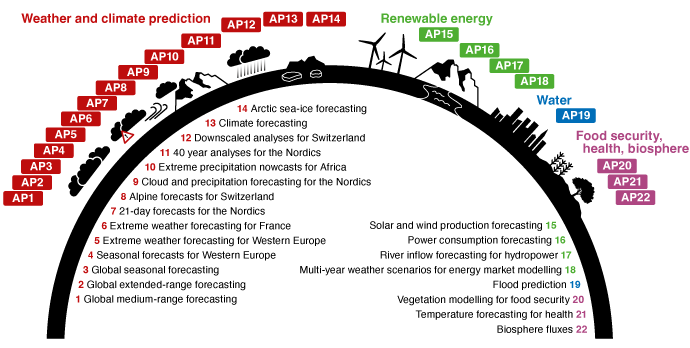
Twenty-two application areas (AP1 to AP22) will be addressed with the WeatherGenerator in the fields of weather and climate prediction; renewable energy; flood prediction; and food security, health and the biosphere.
Many sources of data
The WeatherGenerator will be able to use various different datasets as input, including global and local reanalysis datasets and global and local model output, and also observations from various sources.
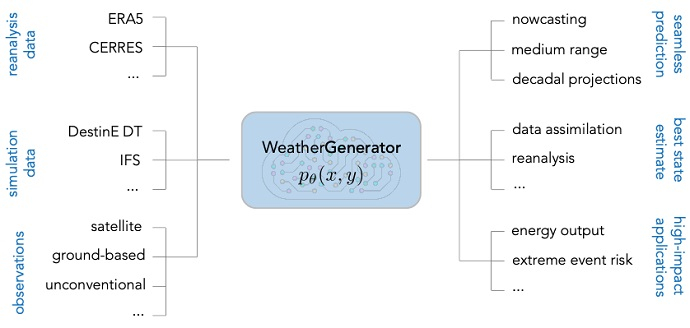
The WeatherGenerator will use many different datasets for training. These include reanalysis datasets, such as ERA5 and CERRES; datasets from weather forecasting and climate prediction systems, such as the DestinE digital twins (DT) and ECMWF’s Integrated Forecasting System (IFS); and observations, including polar-orbiting and geostationary satellites and ground-based observations. With these inputs, the WeatherGenerator can produce a wide range of outputs that serve as a basis for applications.
The conceptual idea of the WeatheGenerator is to let all the data streams come in and to then learn the statistical correlation across the space/time dimensions so that one can be transformed into another:
- If observations are used as inputs and if they are transformed into a (re-)analysis product, the WeatherGenerator is performing data assimilation.
- If (re-)analysis data is used as input and the output is analysis data of a future time, the WeatherGenerator is effectively performing a forecast.
- If model data is used as input and the output is ground-based and local observations at a certain topographic height, the WeatherGenerator is effectively shifting from a grid-cell average to a specific location and therefore performing post-processing.
- If the input is a global and coarse-scale weather model and the output is a local fine-scale weather model, the WeatherGenerator is performing downscaling.
This flexibility allows the WeatherGenerator to be used in many different applications, and also to be more resilient to changes in data ranges and limited training data, when compared to task-specific machine learning applications.
To further enhance the generality of the tool, the outputs can be refined via additional machine learning tools – so-called tail networks – that customise the output of the WeatherGenerator to a specific application.
Masked token modelling
The approach taken to train a machine learning tool that can handle a large number of different inputs is called masked token learning.
In this approach, parts of the data from the input streams are erased (masked) during training. The WeatherGenerator model learns to fill them back in, which requires learning the statistical correlation between the datasets. The results of this learning process can subsequently, for example, be used to fill in missing information if the input streams only cover some data. By masking future information, the training also includes forecasting.
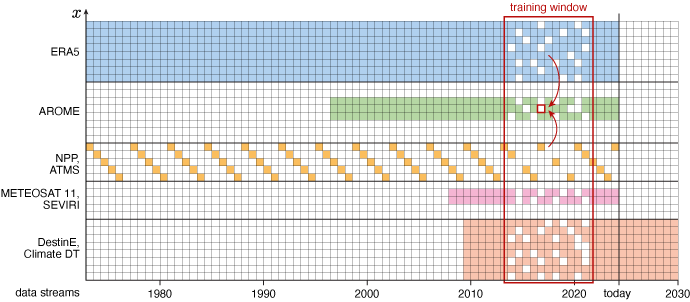
During training, the system is using several streams of input data for a certain area and time period. While some input streams will have some data missing, we are additionally masking out a lot of the incoming data across the streams and the space/time dimensions. The WeatherGenerator will then be trained to recreate the masked data. Once trained, the tool will be able to transform input data into different input streams, and to fill gaps in both space and time.
“To make this work, you need a tool that is much bigger than the AIFS,” says Peter Düben, the Head of ECMWF’s Earth System Modelling Section. “You also need larger datasets, and you need to be able to cope with their diversity. It is not simple, but if it works, then it is likely that it will be better than task-specific models and useful across many different application domains.”
Scope
The WeatherGenerator will have only an atmosphere component (WeGen-Atmo) and a land component (WeGen-Land). This is because most applications are in these two areas.
However, the DestinE initiative will also develop machine learning models for the ocean, waves and sea ice. These will be important, for example, for seasonal predictions. “Whenever we need the ocean, we will link to those tools,” Peter says.
Participants in the project include several European national meteorological services. This will be useful to include limited-area model information. For example, it will be possible to relate global resolutions, as in the global ERA5 reanalysis, to the fine resolutions down to 100 m that are used in local-area modelling and in high-resolution observations.
“In this way, the WeatherGenerator will cover a wide range of scales in space and time, from sub-km to the global domain, and provide a machine learning tool for seamless prediction,” says ECMWF scientist Christian Lessig.
“Very ambitious”
“The WeatherGenerator is a very ambitious project,” Peter says, “but, if it is successful, it will change the way we use machine learning in Earth system applications.”
The result of the WeatherGenerator project will be a generic, open-source European foundation model for weather and climate applications.
The participant organisations are: ECMWF (coordinator); Forschungszentrum Jülich, Earth System Data Exploration Group (Germany); the Norwegian Meteorological Institute; the Max Planck Institute for Biogeochemistry, Department Biogeochemical Integration (Germany); the Royal Netherlands Meteorological Institute; Météo-France; the Swedish Meteorological and Hydrological Institute; the Met Office (UK); the Centro Euro-Mediterraneo sui Cambiamenti Climatici (Italy); the Netherlands eScience Center; Buluttan (Turkey); Kajo Services (Slovakia); Latest Thinking (Germany); Statkraft (Norway); and the associated partners Eidgenössische Technische Hochschule (Switzerland) and MeteoSwiss (Switzerland).
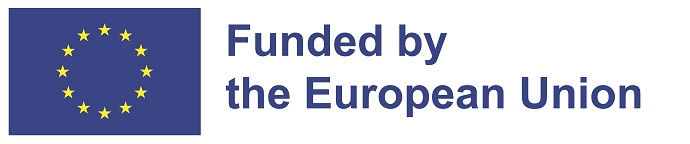
The WeatherGenerator project (grant agreement No. 101187947) is funded by the European Union. Views and opinions expressed are, however, those of the author(s) only and do not necessarily reflect those of the European Union or the Commission. Neither the European Union nor the granting authority can be held responsible for them.